This page is a cumulative notes of mine and can be used as a quick reference of basic definitions in maths that I often encounter on daily basis.
Table of Contents
- Geometric series
- Power series
- Taylor series
- Gradient
- Scalar product
- Vector Norm
- Cauchy-Schwarz inequality
- Law of Cosine
- Orthogonal vectors
- Projection of a vector on a line
- Normalized vector
- Orthonormal vectors
- Orthogonal matrix
- Span the space
- Four fundamental subspaces
- Singular matrices
- Eigenvalues and Eigenvectors
- Matrix rank
- The Gram-Schmidt process
- Linear independence
- Basis for a space
- Orthogonal
- Projection
- Matrix factorisation
Geometric series
Geometric series is a special series where there is a constant ratio between two successive terms. The geometric series can be written in the form,
\[S = \sum_{n=0}^{\infty} ar^{n} = a + ar + ar^2 + ar^3 + ... + ar^n\]where $a$ is a constant. Note that, $S$ is convergent i.i.f $|r| \lt 1$
Example 1:
Given $a = \frac{1}{2}$, $r = \frac{1}{2}$, we have, $$ S_1 = \sum_{n=0}^{\infty} \frac{1}{2} (\frac{1}{2})^n = \frac{1}{2} + \frac{1}{4} + \frac{1}{8} + \frac{1}{16} + ... $$ The ratio between two successive terms in $S_1$ is $\frac{1}{2}$.Example 2:
Given $a = 1$, $r = r$, we have, $$ S_2 = \sum_{n=0}^{\infty} r^n = 1 + r + r^2 + r^3 + ... + r^{n-1} + r^n $$ Multiply both side by $r$, $$ rS_2 = r + r^2 + r^3 + ... + r^n + r^{n+1} $$ Then, $$ S_2 - rS_2 = 1 $$ Thus, $S_2$ converges at $$ S_2 = \frac{1}{1-r} $$References :
Power series
The power series is a series in form of,
\[P = \sum_{n=0}^{\infty} a_n (x - c)^n = a_0 + a_1(x - c) + a_2(x - c)^2 + ... + a_{n-1}(x-c)^{n-1} + a_n(x-c)^{n}\]where $a_n$ is a coefficient of $x_n$, $c$ is a constant
References :
- http://tutorial.math.lamar.edu/Classes/CalcII/PowerSeriesandFunctions.aspx
- https://en.wikipedia.org/wiki/Power_series
Taylor series
Taylor series is a series expansion of a function about a point, i.e., a function $f(x)$ can be approximated about a point $x = a$ by using Taylor series.
If $f(x)$ is differential in $(-\infty, +\infty)$, the Taylor expansion of $f(x)$ about a point $x = a$ has a form,
\[f(x) = \sum_{n=0}^{\infty} \frac{f^{(n)}(a)}{n!} (x - a)^n\]where $f^{(n)}(x)$ is n-th derivative of $f(x)$, e.g., $f^{(1)}(x) = f’(x)$, $f^{(2)}(x) = f’‘(x)$.
Example 1:
Find the Taylor series of $f(x) = e^{-x}$ about $x=0$. We have, $$ \begin{align} f(x) = e^{-x} & \rightarrow f(x = 0) = 1 \\ f'(x) = e^{-x} & \rightarrow f'(x = 0) = -1 \\ f''(x) = e^{-x} & \rightarrow f''(x = 0) = 1 \\ f^{(3)}(x) = e^{-x} & \rightarrow f^{(3)}(x = 0) = -1 \\ & \dots \\ f^{(n)}(x) = (-1)^{n} e^{-x} & \rightarrow f^{(n)}(x = 0) = (-1)^{n} \end{align} $$References:
- https://en.wikipedia.org/wiki/Taylor_series
- http://tutorial.math.lamar.edu/Classes/DE/TaylorSeries.aspx
- http://davidlowryduda.com/an-intuitive-overview-of-taylor-series/
Gradient
The gradient vector $\nabla f$ of a function $f(x_1, x_2, …, x_n)$ is denoted as,
\[\nabla f = \begin{bmatrix} \frac{\partial f}{\partial x_1} & \frac{\partial f}{\partial x_2} & \frac{\partial f}{\partial x_3} \dots \frac{\partial f}{\partial x_n} \end{bmatrix}\]Simply speaking, $\nabla f$ is simply a vector of partial derivatives of $f$.
Example 1:
The $\nabla f$ of the function $f(x, y) = 2x^2y^2 + xy^2$ is $$ \nabla f = \begin{bmatrix} 4xy^2 + y^2 & 4x^2y + 2xy\end{bmatrix} $$References:
Scalar product
The scalar product (also known as dot product, inner product) of two vectors $x = [x_1, x_2, …, x_n]$ and $y = [y_1, y_2, …, y_n]$ is defined as
\[x \cdot y = x^Ty = \sum_{i=1}^{n} x_iy_i = x_1y_1 + x_2y_2 + ... + x_ny_n\]Another way to calculate the scalar product of two vectors $x,y$ is
\[x \cdot y = \lVert x \rVert_2 . \lVert y \rVert_2 . cos \theta\]where $\theta$ is the angle between $x,y$.
Note that scalar product is different to scalar multiplication.
Example 1:
Suppose $x = [1, 2, 3]$, $y = [4, 5, 6]$. We have, $$ x \cdot y = x^Ty = \sum_{i=1}^{3} x_iy_i = 1*4 + 2*5 + 3*6 = 32 $$Alternatively, using R we can have,
> x
[1] 1 2 3
> y
[1] 4 5 6
> sum(x*y)
[1] 32
References:
- http://livebooklabs.com/keeppies/c5a5868ce26b8125/73a4ae787085d554
- http://www.purplemath.com/modules/mtrxmult.htm
Vector Norm
Intuitively speaking, norm of a vector $x = [x_1, x_2, …, x_n]$ is the length of the vector in the space. Norm of the vector $x$ is denoted as $\lVert x \rVert$.
In middle school, the definition of $\lVert x \rVert$ is constraint within the formula $\lVert x \rVert = \sqrt{x_1^2 + x_2^2 + … + x_n^2}$. However, indeed there are numerous ways to calculate the norm and that formula is just one of them.
The norm is universally called as $l_p-norm$. The $l_p-norm$ of $x$ is defined as,
\[\lVert x \rVert_p = \sqrt[p]{\sum_{i=1}^{n} |x_i|^p}\]- Given $p = 2$, we will have $l_2-norm$, which is also known as Euclidean norm. Specifically, we have,
- Given $p=3$, we will have $l_3-norm$, which is, \(\lVert x \rVert_3 = \sqrt[3]{\sum_{i=1}^{n} |x_i|^3} = \sqrt[3]{x_1^3 + x_2^3 + ... + x_n^3}\)
Example 1:
Suppose we have the vector $x = [3, 4, 5]$, the $l_2-norm$ (or Euclidean norm) of $x$ is $$ \lVert x \rVert_2 = \sqrt{\sum_{i=1}^{n} |x_i|^2} = \sqrt{3^2 + 4^2 + 5^2} = 7.071 $$Alternatively, using R, we can have,
> x <- as.matrix(c(3,4,5))
> x
[,1]
[1,] 3
[2,] 4
[3,] 5
> norm(x, "F") #"F" for Euclidean form
[1] 7.071068
References:
- https://rorasa.wordpress.com/2012/05/13/l0-norm-l1-norm-l2-norm-l-infinity-norm/
- https://en.wikipedia.org/wiki/Norm_(mathematics)
Cauchy-Schwarz inequality
For any two vectors $x, y \in \mathbb{R}^n$, we have,
\[x^Ty \leq \lVert x \rVert_2 . \lVert y \rVert_2\]References:
- http://livebooklabs.com/keeppies/c5a5868ce26b8125/73a4ae787085d554
- https://en.wikipedia.org/wiki/Cauchy%E2%80%93Schwarz_inequality
Law of Cosine
The law of cosine (or cosine law) is defined as follows.
Given three vectors $x, y, z \in \mathbb{R}^n$, we have
\[z^2 = x^2 + y^2 - 2 . x. y.cos\theta\]where $\theta$ is the angle between vectors $x, y$.
References:
Orthogonal vectors
Given two vectors $x, y \in \mathbb{R}^n$, $x,y$ are orthogonal i.i.f the scalar product of $x,y$ equals 0. Mathematically, we have,
\[x \perp y \leftrightarrow x \cdot y = x^Ty = 0\]Projection of a vector on a line
The explanation is given in this video.
References:
Normalized vector
The normalized vector (or unit vector) $\hat{x}$ of the vector $x \in \mathbb{R}^n$ is a vector whose norm equals to 1 and has the same direction with $x$. Mathematically, we have,
\[\hat{x} = \frac{x}{\lVert x \rVert_p}\]By using R, we can have,
> x <- c(1,2,3,4,5,6)
> x
[1] 1 2 3 4 5 6
> x / sqrt(sum(x^2)) # Using Euclidean norm
[1] 0.1048285 0.2096570 0.3144855 0.4193139 0.5241424
[6] 0.6289709
References:
Orthonormal vectors
Two vectors $q_1, q_2 \in \mathbb{R}^n$ are orthonormal if they are:
- Unit vectors (or normalized vectors), e.g., $q_1 = \frac{x}{\lVert x \rVert_p}$, and $q_2 = \frac{y}{\lVert y \rVert_p}$ .
- Orthogonal, e.g., $q_1^Tq_2 = 0$.
By using R, we can have,
> x <- c(1,2,3,4)
> x
[1] 1 2 3 4
> y <- c(3,2,3,-4)
> y
[1] 3 2 3 -4
> x%*%y #vectors x, y are orthogonal
[,1]
[1,] 0
> x_hat <- x/sqrt(sum(x^2)) #unit vector of x
> x_hat
[1] 0.1825742 0.3651484 0.5477226 0.7302967
> y_hat <- y/sqrt(sum(y^2)) #unit vector of y
> y_hat
[1] 0.4866643 0.3244428 0.4866643 -0.6488857
> x_hat%*%y_hat #x_hat, y_hat are orthonormal vectors
[,1]
[1,] 5.551115e-17
References:
Orthogonal matrix
An orthogonal matrix $Q$ is simply a square matrix with orthonormal columns. Mathematically, we have,
\[Q = \begin{bmatrix} q_1 & q_2 & \dots & q_n \end{bmatrix}\]where $q_i$ is orthonormal vector.
Note that $Q^TQ = I$
> x <- c(0,0,1)
> y <- c(1,0,0)
> z <- (0,1,0)
> x%*%y #vectors x,y are orthonormal
[,1]
[1,] 0
> x%*%z #vectors x,z are orthonormal
[,1]
[1,] 0
> y%*%z #vectors y,z are orthonormal
[,1]
[1,] 0
> Q <- matrix(c(x,y,z), nrow = 3, byrow = FALSE) #Q is orthogonal matrix
> Q #Q is a permutation matrix
[,1] [,2] [,3]
[1,] 0 1 0
[2,] 0 0 1
[3,] 1 0 0
> t(Q) #transpose of Q
[,1] [,2] [,3]
[1,] 0 0 1
[2,] 1 0 0
[3,] 0 1 0
> Q%*%t(Q) #Q^T*Q = I
[,1] [,2] [,3]
[1,] 1 0 0
[2,] 0 1 0
[3,] 0 0 1
References:
- Linear Algebra and Its Application, Gilbert Strang, 3rd Edition.
Span the space
Vectors $v_1, …, v_n$ span a space $V$ means that the space consists of all combinations of these vectors. Another way to understand is that __we can express any random vector $w$ in the vector space $V$ by a linear combination of the vectors $v_i$, e.g., $w = c_1v_1 + c_2v_2 + … + c_nv_n$.
Mathematically, we have the definition of span.
\[span(v_1, v_2, ..., v_n) = \{c_1v_1 + c_2v_2 + ... + c_nv_n \}\]Example 1:
Given the matrix $$A = \begin{bmatrix} 1 & 6 \\ 2 & 7 \\ 3 & 8 \end{bmatrix}$$, the column space of the matrix $A$ is defined by the span of the column vectors $$v_1 = \begin{bmatrix} 1 \\ 2 \\ 3 \end{bmatrix}$$ and $$v_2 = \begin{bmatrix} 6 \\ 7 \\ 8 \end{bmatrix}$$ as follows. $$ \begin{align} C(A) & = span(c_1, c_2) \\ & = c_1\begin{bmatrix} 1 \\ 2 \\3 \end{bmatrix} + c_2\begin{bmatrix} 6 \\ 7 \\ 8 \end{bmatrix} \end{align} $$References:
Four fundamental subspaces
There are four fundamental subspaces of a matrix
- The column space of the matrix $A$, denoted by $C(A)$ The column space of a matrix is defined by the span of all column vectors $c_i$.
where $c_i \in \mathbb{R}^{mxn}$ is the column vectors of the matrix $A$, $w_i \in \mathbb{R}$ is the coefficients.
-
The nullspace of the matrix $A$, denoted by $N(A)$ The null space of a matrix contains all vectors $x$ such that $Ax = 0$.
- The row space of the matrix $A$, denoted by $R(A)$ The row space $R(A)$ is the column space of $A^T$. Thus, $R(A) = C(A^T)$.
- The left nullspace of the matrix $A$ The left null space of $A$ is the nullspace of $A^T$.
Example:
Suppose we have the matrix \(A = \begin{bmatrix} 1 & 1 & 1 & 1 \\ 1 & 2 & 3 & 4 \\ 4 & 3 & 2 & 1 \end{bmatrix}\), we have,
1.The column space $C(A)$
\[\begin{align} C(A) & = span(c_1, c_2, c_3, c_4) \\ & = w_1\begin{bmatrix} 1 \\ 1 \\ 4 \end{bmatrix} + w_2\begin{bmatrix} 1 \\ 2 \\ 3 \end{bmatrix} + w_3\begin{bmatrix} 1 \\ 3 \\ 2 \end{bmatrix} + w_4\begin{bmatrix} 1 \\ 4 \\ 1 \end{bmatrix} \end{align}\]Let’s say $w_1 = 2, w_2 = 3, w_3 = 1, w_4 = 1$, we have a vector
\(\begin{align} v = 2\begin{bmatrix} 1 \\ 1 \\ 4 \end{bmatrix} + 3\begin{bmatrix} 1 \\ 2 \\ 3 \end{bmatrix} + 1\begin{bmatrix} 1 \\ 3 \\ 2 \end{bmatrix} + 1\begin{bmatrix} 1 \\ 4 \\ 1 \end{bmatrix} = \begin{bmatrix} 7 \\ 15 \\ 20 \end{bmatrix} \end{align}\).
Since $v$ is the result of the linear combination, \(v = \begin{bmatrix} 7 \\ 15 \\ 20 \end{bmatrix}\) is in the column space $C(A)$.
2.The nullspace $N(A)$
\[Ax = 0 \\ \rightarrow \begin{bmatrix} 1 & 1 & 1 & 1 \\ 1 & 2 & 3 & 4 \\ 4 & 3 & 2 & 1 \end{bmatrix} \begin{bmatrix} x_1 \\ x_2 \\ x_3 \\ x_4 \end{bmatrix} = 0 \\ \begin{align} \rightarrow x_1 & = x_3 + 2x_4 \\ x_2 & = -2x_3 - 3x_2 \end{align}\]Thus,
\[\begin{bmatrix} x_1 \\ x_2 \\ x_3 \\ x_4 \end{bmatrix} = x_3\begin{bmatrix} 1 \\ -2 \\ 1 \\ 0 \end{bmatrix} + x_4\begin{bmatrix} 2 \\ -3 \\ 0 \\ 1 \end{bmatrix}\]Finally, we have,
\[N(A) = span(x_3, x_4) = x_3\begin{bmatrix} 1 \\ -2 \\ 1 \\ 0 \end{bmatrix} + x_4\begin{bmatrix} 2 \\ -3 \\ 0 \\ 1 \end{bmatrix}\]References:
- Linear algebra and its application, Gilbert Strang, 3rd Edition.
- https://www.khanacademy.org/math/linear-algebra/vectors-and-spaces/null-column-space/v/null-space-2-calculating-the-null-space-of-a-matrix
Singular matrices
Singular matrix is a square matrix which does not have an inverse. A square matrix is singular iif its determinant is zero.
For example:
\[\begin{bmatrix} 2 & 6 \\ 1 & 3 \end{bmatrix}\]is singular since
\[\begin{vmatrix} 2 & 6 \\ 1 & 3 \end{vmatrix} = 2*3 - 6*1 = 0\]Geometric interpretation A matrix can be thought of as a linear function from a vector space $V$ to a vector space $W$. Typically, one is concerned with $n×n$ real matrices, which are linear functions from $ℝ^n$ to $ℝ^n$. An $n×n$ real matrix is non-singular if its image as a function is all of $ℝ^n$ and singular otherwise. More intuitively, it is singular if it misses some point in $n$-dimensional space and non-singular if it doesn’t.
References:
- Linear algebra and its application, Gilbert Strang, 3rd Edition.
- http://math.stackexchange.com/questions/166021/what-is-the-geometric-meaning-of-singular-matrix
Eigenvalues and Eigenvectors
Suppose we have a matrix $A$ that acts like a function, when it would takes a vector $x$ and output a vector $Ax$. At this moment, we are more interested in vectors that come out in the same direction as they went in. That is not typical and there will be only a few vectors $Ax$ that are parallel to $x$. Those are called eigenvectors. It can be expressed by
\[\mathbf{A}x = \lambda x\]where we would call $\lambda$ the eigenvalue and $x$ the eigenvector.
**How to solve $Ax = \lambda x$
Step 1. Rewrite: $(A - \lambda I)x = 0$
We can see that the matrix $A - \lambda I$ must be singular otherwise, $x$ is a zero matrix, which is not interesting at all!
Proof: Suppose the matrix $T = A - \lambda$, thus we have
\[T x = 0\]Multiply both side of the equation by $T^{-1}$, we have
\[T^{-1}Tx = 0\]If $T$ is a singular matrix, then
\[T^{-1}Tx = 0 \\ \Leftrightarrow Ix = 0 \\ \Leftrightarrow x = 0\]We conclude that if $T$ is singular, then $x = 0$, which is too obvious that it is not interesting at all!
Step 2. Because $A - \lambda I$ is a singular matrix, we would have
\[det(A - \lambda I) = 0\]By computing the determinant of $A - \lambda I$, we can find the eigenvalue $\lambda$.
Step 3. For each eigenvalue, solve the equation $(A - \lambda I) x = 0$ to find eigenvector $x$.
References:
- Linear algebra and its application, Gilbert Strang, 3rd Edition.
Matrix rank
The rank of matrix equals to the maximum number of independent columns or the maximum number of independent row.
- Every matrix of rank one has the simple form $A = uv^T$
Example 1:
The matrix $$ A = \begin{bmatrix} 1 & 0 & 1 \\ -2 & -3 & 1 \\ 3 & 3 & 0 \end{bmatrix} $$ has rank 3 because 3 rows are linearly independent.Example 2:
The matrix $$ B = \begin{bmatrix} 1 & 1 & 0 & 2 \\ -1 & -1 & 0 & -2 \end{bmatrix} $$ has rank 1 because two rows are linearly dependent.References:
- https://en.wikipedia.org/wiki/Rank_(linear_algebra)
The Gram-Schmidt process
The Gram-Schmidt process is a method for orthonormalising a set of vectors.
Linear independence
Vectors $v_1, …, v_k$ are linearly independent if $ c_1 v_1 + … + c_k v_k = 0$ only happens when $c_1 = c_2 = … = c_k = 0$.
Example 1:
The columns of matrix $$ A = \begin{bmatrix} 1 & 3 & 3 & 2 \\ 2 & 6 & 9 & 5 \\ -1 & -3 & 3 & 0 \end{bmatrix} $$ are linearly dependent, because the 2nd column is 3 times the 1st column.If the columns are linearly independent, the nullspace contains only zero vectors.
References:
- Linear algebra and its application, Gilbert Strang, 3rd Edition.
Basis for a space
Basis for a space is a sequence of vectors $v_1, v_2, …, v_d$ with 2 properties:
- They are independent.
- They span the space.
It means that every vector in the space is a combination of the basis vector because basis vectors span the space.
Example 1:
Space $\mathbb{R}^3$ has a basis is $$ \begin{bmatrix} 1 \\ 0 \\ 0 \end{bmatrix} \begin{bmatrix} 0 \\ 1 \\ 0 \end{bmatrix} \begin{bmatrix} 0 \\ 0 \\ 1 \end{bmatrix} $$ because (1) they are linearly independent (2) we can express any vector in $\mathbb{R}^3$ by these 3 vectors.Example 1:
Space $\mathbb{R}^3$ has a basis is $$ \begin{bmatrix} 1 \\ 1 \\ 2 \end{bmatrix} \begin{bmatrix} 2 \\ 2 \\ 5 \end{bmatrix} $$ because (1) they are linearly independent (2) we can express any vector in $\mathbb{R}^3$ by these 3 vectors.References:
- Linear algebra and its application, Gilbert Strang, 3rd Edition.
Orthogonal
Subspace $S$ is orthogonal to subspace $T$ means every vector in $S$ is orthogonal to every vector in $T$.
** It is important to remember that a plane cannot be orthogonal to a plan.
A typical example is that the wall and the floor of a room look like perpendicular planes in $\mathbb{R}^3$. But by the definition of algebra, it is not so. Because the common line in both the wall and the floor cannot be orthogonal to itself.
1. The row space is orthogonal to the null space & the column space is orthogonal to the left nullspace.
Proof: Suppose $x$ is the vector in the null space. Thus, we have $Ax = 0$.
\[Ax = 0 \\ \leftrightarrow \begin{bmatrix} \cdots & row 1 & \cdots \\ \cdots & row 2 & \cdots \\ \cdots & \cdots & \cdots \\ \cdots & row 3 & \cdots \end{bmatrix} \begin{bmatrix} x_1 \\ x_2 \\ x_3 \\ \cdots \\ x_n \end{bmatrix} = \begin{bmatrix} 0 \\ 0 \\ 0 \\ \cdots \\ 0 \end{bmatrix}\]It means that we have $row 1 \cdot x = 0$ $\Rightarrow$ row 1 is orthogonal to $x$.
2. The space off all vectors orthogonal to a subspace $V$ is called the orthogonal complement of $V$, and denoted by $V^{\perp}$.
As a result, the row space and the nullspace are orthogonal complements.
Projection
1. The cosine of the angle between any two vectors $a$ and $b$ is
\[cos \, \theta = \frac{a^T b}{\lVert a \rVert \lVert b \rVert}\]Proof: Using the law of cosine, we have,
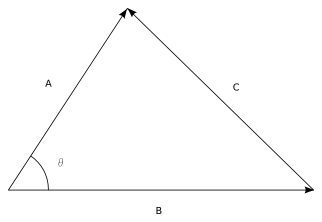
The cosine of the angle between two vectors $a$ and $b$
2. The projection of $b$ onto $a$ is
\[p = xa = \frac{a^Tb}{aTa}a\]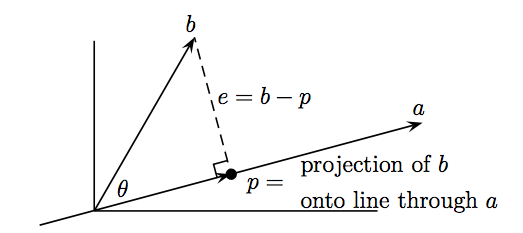
The projection of $b$ onto $a$ where $p = xa$
Proof: we have $p = xa$ (because $p$ is on $a$), thus we have the vector $e = b - p$.
Because $e$ is orthogonal to $a$, we can have $a^Te = 0$.
\[\begin{align} &\, a^Te = 0 \\ &\Leftrightarrow a^T(b -p) = 0 \\ &\Leftrightarrow x = \frac{a^Tb}{a^Ta} \\ &\Rightarrow p = \frac{a^Tb}{a^Ta}a \label{projection_matrix} \end{align}\]3. The projection matrix $\mathbf{P}$ is the matrix that project $b$ onto $a$
\[\begin{align} \mathbf{P} = a \frac{a^Tb}{a^Ta} \label{projection_matrix_definition} \end{align}\]Proof: We already have in (\ref{projection_matrix}) that $p = \frac{a^Tb}{a^Ta}a$, by rewriting
\[\begin{align} p &= \frac{a^Tb}{a^Ta}a \\ \Leftrightarrow p &= \frac{a a^T}{a^Ta} b \\ \Leftrightarrow p &= \mathbf{P}b \end{align}\]Matrix factorisation
1. Factorisation $A = QR$
We can factorise any square matrix $A$ as
\[A = QR\]where $Q$ is an orthogonal matrix, $R$ is an upper triangular matrix.
Example 1:
$$ A = \begin{bmatrix} 1 & 1 & 2 \\ 0 & 0 & 1 \\ 1 & 0 & 0 \end{bmatrix} = \begin{bmatrix} \frac{1}{\sqrt{2}} & \frac{1}{\sqrt{2}} & 0 \\ 0 & 0 & 1 \\ \frac{1}{\sqrt{2}} & -\frac{1}{\sqrt{2}} & 0 \end{bmatrix} \begin{bmatrix} \sqrt{2} & \frac{1}{\sqrt{2}} & \sqrt{2} \\ & \frac{1}{\sqrt{2}} & \sqrt{2} \\ & & 1 \end{bmatrix} $$> x <- matrix(data = c(1, 1, 2, 0, 0, 1, 1, 0, 0), nrow = 3, ncol = 3, byrow = T)
> x
[,1] [,2] [,3]
[1,] 1 1 2
[2,] 0 0 1
[3,] 1 0 0
> qr(x)
$qr
[,1] [,2] [,3]
[1,] -1.4142136 -0.7071068 -1.414214
[2,] 0.0000000 -0.7071068 -1.414214
[3,] 0.7071068 -1.0000000 1.000000
$rank
[1] 3
$qraux
[1] 1.707107 1.000000 1.000000
$pivot
[1] 1 2 3
attr(,"class")
[1] "qr"
2. Factorisation $A = LU$
LU decomposition simply refers to the transformation of the square matrix $A$ into the product of two different matrices $L$ (lower triangular matrix) and $U$ (upper triangular matrix). Mathematically, we have $A = LU$.
Example 1:
$$ \begin{align} A = \begin{bmatrix} 1 & 2 & 3 \\ 4 & 5 & 6 \\ 7 & 8 & 9 \end{bmatrix} & = LU \\ & = \begin{bmatrix} 1 & 0 & 0 \\ 4 & 1 & 1 \\ 7 & 2 & 2 \end{bmatrix}\begin{bmatrix} 1 & 2 & 3 \\ 0 & -3 & -6 \\ 0 & 0 & 0 \end{bmatrix} \end{align} $$By using R, we can also have,
> library(pracma)
> x <- matrix(c(1, 2, 3, 4, 5, 6, 7, 8, 9), nrow = 3, byrow = TRUE)
> x
[,1] [,2] [,3]
[1,] 1 2 3
[2,] 4 5 6
[3,] 7 8 9
> lu(x)
$L
[,1] [,2] [,3]
[1,] 1 0 0
[2,] 4 1 0
[3,] 7 2 1
$U
[,1] [,2] [,3]
[1,] 1 2 3
[2,] 0 -3 -6
[3,] 0 0 0
3. Factorisation $A = S \Lambda S^{-1}$
Given the eigenvector matrix $S$, which has the form $S = \begin{bmatrix} x_1 & x_2 & \cdots & x_n \end{bmatrix}$, where $x_1, …, x_n$ are the eigenvectors of any square matrix $A$ with distinct eigenvalues $\lambda_1, …, \lambda_n$, we can use $S$ to diagonalise A mathematically by
\[\begin{align} AS = S \Lambda \end{align}\]where $\Lambda$ is the eigenvalue matrix, which has the form \(\Lambda = \begin{bmatrix} \lambda_1 & & & \\ & \lambda_2 & & & \\ \ & & \ddots & \\ & & & \lambda_n \end{bmatrix}\)
From that we can also have
\[\begin{align} AS = S \Lambda \quad \text{or} \quad A = S \Lambda S^{-1} \end{align}\]> A <- matrix(data = c(2, 1, 0, 0), nrow = 2, ncol = 2, byrow = T) # Define the matrix
> A
[,1] [,2]
[1,] 2 1
[2,] 0 0
> E <- eigen(A) # Find eigenvectors and eigenvalues of A
> S <- E$vectors # The eigenvector matrix S
> Lambda <- diag(E$values) # The eigenvalue matrix Lambda
> S_inv <- solve(S)
> A <- S %*% Lambda %*% S_inv # Confirm if A = S*Lambda*S_inv
> A
[,1] [,2]
[1,] 2 1
[2,] 0 0
4. Factorisation $A = Q \Lambda Q^T$
Given the factorisation $A = S \Lambda S^{-1}$, if matrix $A$ is symmetric, we can have
\[A = S \Lambda S^{-1} = Q \Lambda Q^T\]where $S$ is the eigenvector matrix, $\Lambda$ is the eigenvalue matrix, $Q$ is the orthogonal matrix.
We can see that when $A$ is symmetric, the orthogonal matrix $S$ can be the orthogonal matrix $Q$ (which also mean eigenvectors are orthogonal to each other).
> A <- matrix(data = c(2, -1, -1, 2), nrow = 2, ncol = 2, byrow = T) # Matrix A
> A
[,1] [,2]
[1,] 2 -1
[2,] -1 2
> E <- eigen(A) # Find eigenvectors and eigenvalues of A
> S <- E$vectors # The eigenvector matrix S
> Lambda <- diag(E$values) # The eigenvalue matrix Lambda
> S_inv <- solve(S)
> A <- S %*% Lambda %*% S_inv # Confirm if A = S*Lambda*S_inv
> A
[,1] [,2]
[1,] 2 -1
[2,] -1 2
> t(S) %*% S # Check if S is orthogonal matrix
[,1] [,2]
[1,] 1.000000e+00 -2.237114e-17
[2,] -2.237114e-17 1.000000e+00
5. Factorisation $A = Q_1 \Sigma Q_2^T$
Any $m x n$ matrix $A$ can be factored into
\[A = Q_1 \Sigma Q_2^T\]The columns of $Q_1$ ($m$ by $m$) are eigenvectors of $AA^T$, and the columns of $Q_2$ ($n$ by $n$) are eigenvectors of $A^TA$. The $r$ singular values on the diagonal of $\Sigma$ ($m$ by $n$) are the square roots of the nonzero eigenvalues of both $AA^T$ and $A^TA$.
References: